Software
Packages:
- SELM: Fluctuating Hydrodynamics. Fluid-structure interaction simulations for soft materials and complex fluids using fluctuating hydrodynamics approaches (interfaces with LAMMPS MD package) [github] [tutorials/documentation].
- GNP: Geometric Neural Operators. Geometric Neural Operators (GNPs) for machine learning tasks on point-cloud representations: curvature estimation, shape deformation, solvers for geometric PDEs, training transferable geometric foundation models, and other tasks [github].
- MLMOD: Machine Learning Simulation Methods. Machine learning package for data-driven modeling and simulation of particle systems, materials, and complex fluids (interfaces with LAMMPS MD package) [github] [documentation pages].
- GD-VAEs: Geometric Dynamic Variational Autoencoders. Machine learning package for variational autoencoders (vae's) for learning general representations and non-linear dynamics in manifold latent spaces incorporating topological and geometric information [github].
- GMLS-Nets: Generalized Moving Least Squares Approaches. Machine learning methods for operator regression on unstructured data for (ODEs/PDEs/reduced-order models/...) [github].
For additional packages and codes, see our github repositories.
Scientific Computation
Mango-Selm Package : Fluid-Structure Interaction Simulations and Fluctuating Hydrodynamics for LAMMPS (USER-SELM), Stochastic Immersed Boundary Methods / SELMs
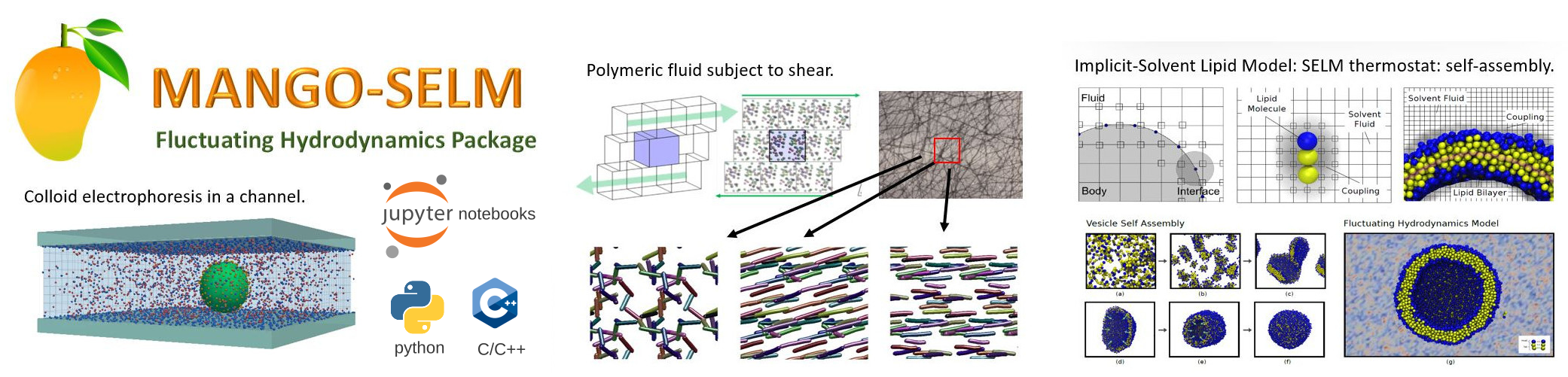
Package for performing fluid-structure interaction simulations. Integrated with LAMMPS, an optimized molecular dynamics package for modeling mechanical systems and provides many types of interaction force laws, model types, and statistical analysis tools. The package was motivated by implicit-solvent coarse-grained simulations in molecular dynamics, and for more general problems involving fluid-structure interactions subject to thermal fluctuations. The package allows for handling fluid-structure interactions using stochastic continuum fluid equations. Deterministic simulations are also possible by setting temperature parameter to zero in models.
Python and Jupyter notebook interfaces are also now available.
Quick Start
To install pre-compiled package for Python use
pip install -U selm-lammps
To test the package installed run
python -c "from selm_lammps.tests import t1; t1.test()"
Pre-compiled binaries for (Debian 9+/Ubuntu and Centos 7+, Python 3.6+). Also works on Windows using WSL Ubuntu 22.04.
GitHub: Download examples and additional information here.
MLMOD-PYTORCH: Machine Learning Methods for Data-Driven Modeling and Simulation in Particle Systems, Materials, and Fluids (LAMMPS)
Python and Jupyter notebook interfaces are also now available.
Quick Start
To install pre-compiled package for Python using pip download package below.
MLMOD: Machine Learning Methods for Data-Driven Modeling in LAMMPS, P. J. Atzberger, (2022), [preprint] [arXiv] [video: talk overview]
GitHub: download software and examples here
GNP-PYTORCH: Geometric Neural Operators (GNPs) Package
Python and Jupyter notebook examples are also now available.
Quick Start
Please see
GitHub: install instructions and examples here
Stochastic Immersed Boundary Method
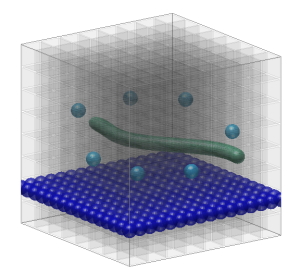
Stochastic Immersed Boundary Methods provide approaches for fluid-structure interactions subject to thermal fluctuations. An implementation and tutorial for how to use these methods is provided here:
Downloads and additional information here.
Machine Learning
GD-VAEs : Geometric Dynamic Variational Autoencoders
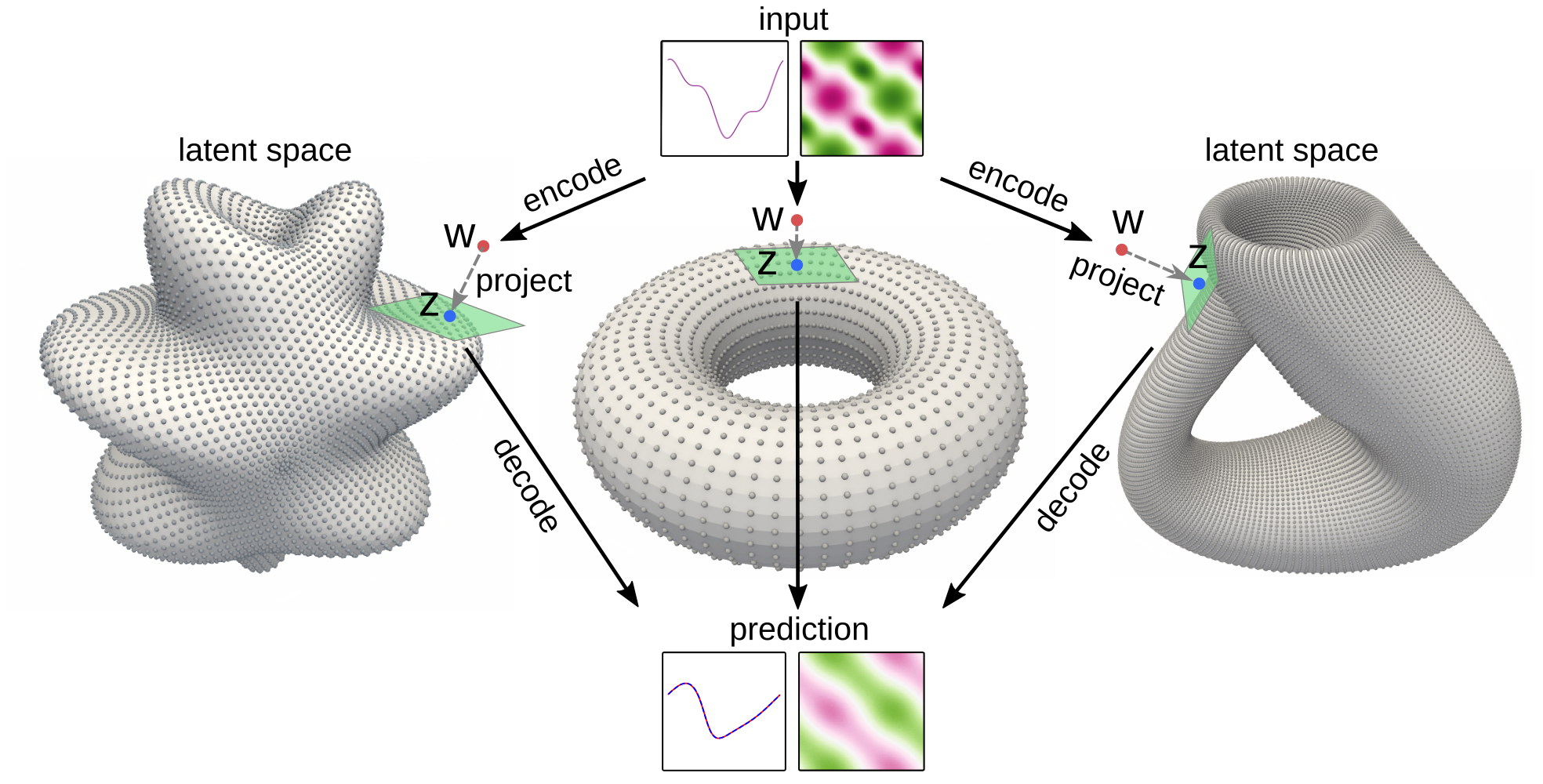
Geometric Dynamic Variational Autoencoders (GD-VAE) package provides machine learning methods for learning embedding maps for nonlinear dynamics into general latent spaces. This includes methods for standard latent spaces or manifold latent spaces with specified geometry and topology. The manifold latent spaces can be based on analytic expressions or general point cloud representations.
Methods and codes related to the paper:
GD-VAEs: Geometric Dynamic Variational Autoencoders for Learning Nonlinear Dynamics and Dimension Reductions, R. Lopez and P. J. Atzberger, (preprint), (2022), [preprint] [graphical abstract] [arXiv] [software].
Python and Jupyter notebook interfaces.
Quick Start
To install pre-compiled package for Python use
pip install -U gd-vae-pytorch
To test the package installed run
python -c "import gd_vae_pytorch.tests.t1 as t1; t1.run()"
GitHub: download software and examples here
GMLS-Nets
[software] [documentation] [examples]
Download Latest Release [git-hub page]
GMLS-Nets: A Framework for Learning from Unstructured Data, N. Trask, R. G. Patel, B. J. Gross, and P. J. Atzberger, (accepted), [preprint] [arXiv].
Git-Hub: Additional Projects and Information
- course materials
Course Supplemental Materials
Data Science and Machine Learning
Image Classification using Convolutional Neural Networks (course exercise)
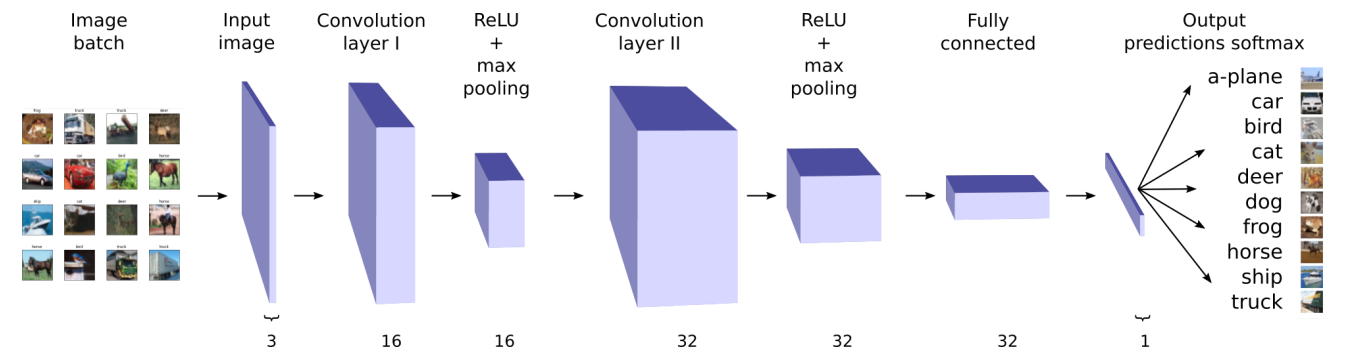
Jupyter Notebook Codes | CIFAR10 PDF | MNIST PDF | Data Folder
Facial Recognition and Feature Extraction (course exercise)
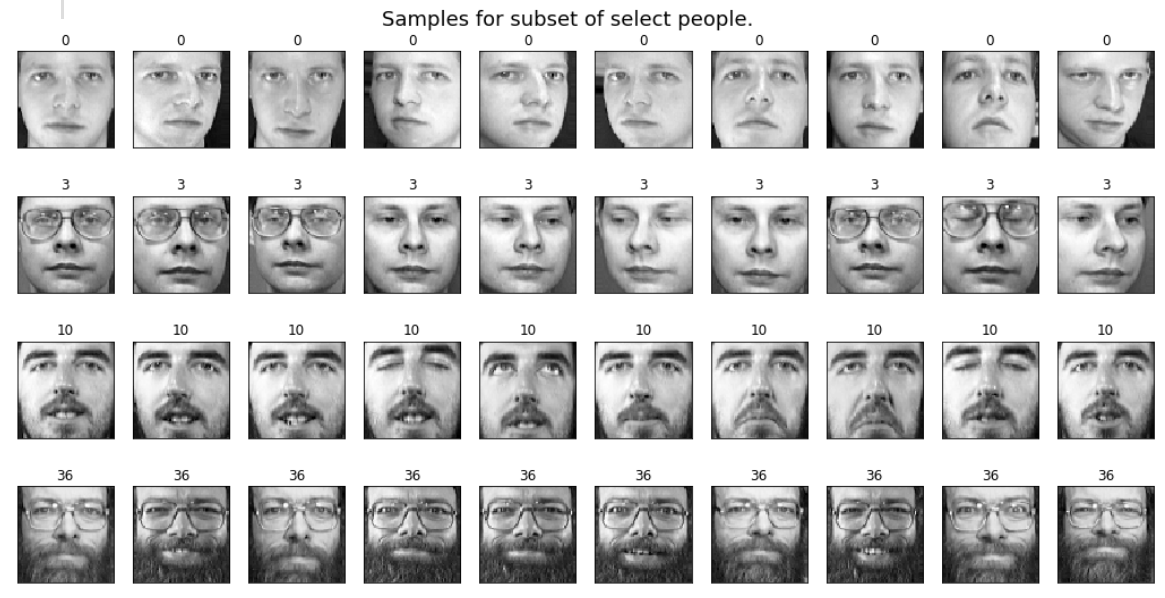
Jupyter Notebook Codes | Jupyter PDF | Data Folder | Kaggle: Facial Recognition (SVM) | Kaggle PDF
Machine Learning
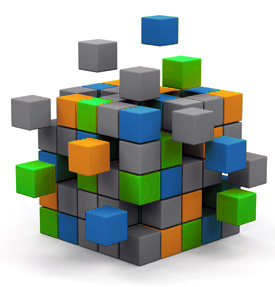
Machine Learning: Foundations and Applications Course (MATH CS 120)
[Course Materials]